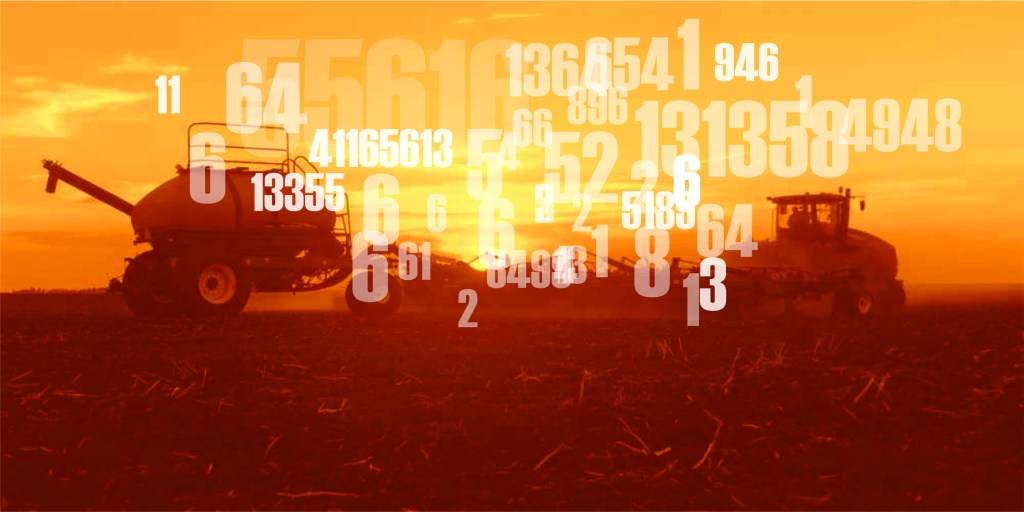
Crop disease or insect pest outbreaks can be difficult to predict. Agronomists and gov extension peoples put out collection traps, analyze the weather and make forecasts for the upcoming season. What if we incorporated sales data of crop protection products by geography from the previous year to enhance those predictions? This is only one example of how Big Data can benefit the crop production industry.
Everyone has heard of the term “Big Data.” In this day and age of smartphones, electronic transactions and the internet of things, everyone is connected, and large sums of data are being generated. Transactions, purchases, social media, search histories, personal interests, etc. When all this data is aggregated it can be used to predict buying trends, consumer preferences, human behavior. As an example, it is said that social media may now be a better/faster predictor of potential disease outbreaks than cases reported through traditional health channels.
What does Big Data mean for Agriculture?
Big Data is promising to revolutionize agriculture and, more specifically, crop production. By aggregating the reams of data collected through modern farming technology we can better analyze field variability and compare management practices. Everything from elevation maps to yield, moisture, application maps and even fuel consumption can all be used as layer to better understand the productivity of our fields. Couple this with the data generated from an infinite number of farms and we can start to identify trends in which combination of inputs produce the best returns. We can maximize the potential of every acre, fit the right varieties on the right fields, minimize over application and environmental losses.
So, what is the Problem?
Right now, the biggest challenge or bottleneck in the adoption of Big Data in crop production is collecting quality data. There has been a large injection of venture capital money into developing the tools to make big data work because there is a potential for big payoffs. Unfortunately, there are too many variables that need to be addressed before we can really make it work.
Unpredictable Weather
The biggest variable right now is the unpredictability of the weather. We are at the mercy of mother nature and she likes to keep us guessing. Weather will make or break your season no matter how well you plan or how good of a start it gets. Weather can vary from one end of a field to the other never mind trying tot make sense of it over wide geographies. Weather has been influencing the development of our soils for millenniums before we even started farming it. You can barely compare farms 50 miles apart, never mind in the next state or province over. Big Data requires a certain level of predictability. It works great in controlled setting like retail stores. It plays a big role in dairy and hog production where most aspects can be controlled from genetics to feed, temperature, and how long you leave the lights on.
I believe that before “Big Data” can work, we need to figure out the “Small Data”
Garbage Data In / Garbage Data Out
The next bottleneck or problem in collecting quality crop production data is in the equipment itself. Current yield monitor technologies in harvesters are notoriously difficult to calibrate and require constant maintenance and adjustment. This leads to incorrect yields and incomplete maps. Most farming operations run multiple harvesters that all need to be calibrated similarly. The silver lining is that new generation yield monitors are self calibrating and harvest machines can now link up and feed data to the cloud resulting in better quality data.
As companies race to sign up acres there are many that claim they can make bad data good, but we still have a long way to go. We have lots to learn about how to best capture field and weather variability. There are many companies developing tools that will carry us into the next phases of production agriculture but until then, how do we address current shortcomings?
Small Data before Big Data
I believe that before “Big Data” can work, we need to figure out the “Small Data”. When I say small data, I really mean smaller geographies. By comparing data production data between 10 to 20 farm operations in say a 10 to 20 mile radius we can better compare apples to apples. Small Data will be led by a local agronomy group that understands the local soils and growing environments. Producers have in-depth knowledge of their own operations but historically have been reluctant to share ideas with neighbors. Agronomists on the other hand have in-depth knowledge of numerous operations and are well positioned interpret the data and offer additional insights. A 3rd party is often necessary to act as gatekeepers in collecting and aggregating the data.
Its this type of peer group learning that will help producers grow their operations faster and farther than trying to make a go of it alone. Change is constant, it is inevitable, and it will only come faster with the adoption of machine learning and Big Data. There are still producers alive today (hopefully retired) that started their farming careers in the days of horse drawn plows. I am convinced we will see more changes in the next 10 years than we saw in the last 30.